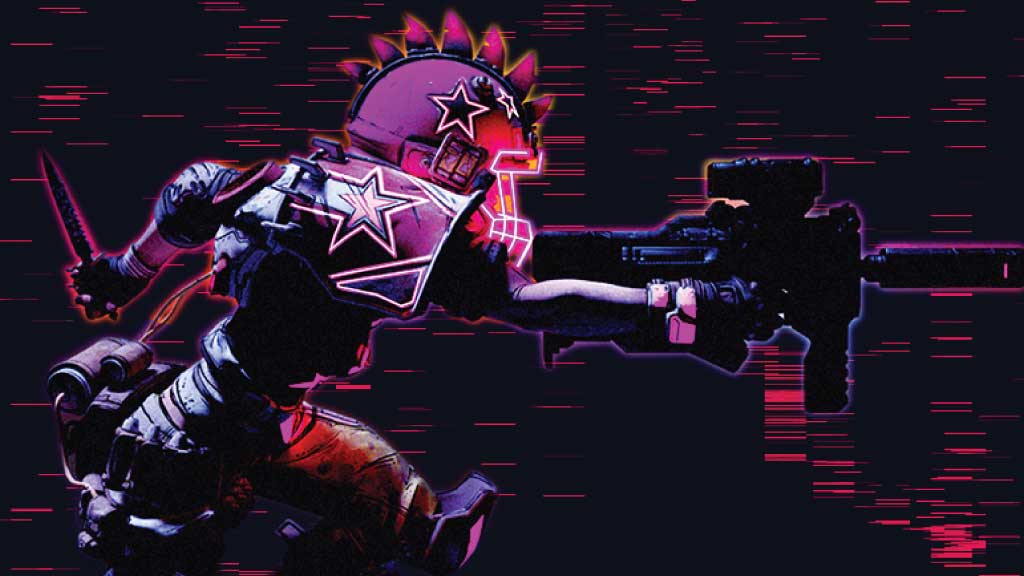
07 May BORDERLANDS SCIENCE UPDATE : A PEEK AT THE DATA
In our last blog post, we described high level ideas and answered commonly asked questions about how and why Borderlands Science works. We also encouraged you to reach out to us via our subreddit to ask questions, and many of the questions we received touched the data, what it would look like and how we could use it. We decided to share some bite-sized insight on the topic. Keep in mind the project just started and a lot of science will have to be done to take advantage of this data, so what we are sharing today is more akin to observations than results.
Do players actually go further than the target score?
The most common comment we received was that there was not a lot of in-game incentive to go beyond the target score, and that it would be possible to simply make an AI play the game to collect this type of data. In fact, most players provide solutions that are quite distinct, both from what a program gives us, and between players. To back up this statement, we extracted data from 40,000 pareto-optimal solutions (i.e. solutions that are not strictly worse than any other solution) that were specific to a small, 5-nucleotide long region of the genome we are looking at.
How different are the players’ solutions from the computer’s solutions?
For each bacterial DNA sequence that was tested on this small region, we extracted all the puzzles solutions that involved this sequence and compared the position of that sequence’s nucleotides (or bricks) with the computer’s alignment. Then, for each sequence, we compute the average similarity between the computer’s solution and the players’. If a sequence has a similarity of 1, it means that in every puzzle solution, the players’ answer was identical to the computer’s. A similarity of 0 means the two solutions have nothing in common.
We show the results of this investigation in the following plot. Here, each horizontal line is a sequence. The blue region is the part of the sequence that is, on average, identical to the computer’s solution, and the orange region is the part that is distinct. Overall, 38% of the total sequence content diverges from the computer. Remember that to submit a puzzle, you need to at least equalize with the computer (the target score). This means that on average, a player submitted a solution in which ~60% of the bricks were where the computer put them, and 40% were positioned better, and that is a significant improvement.
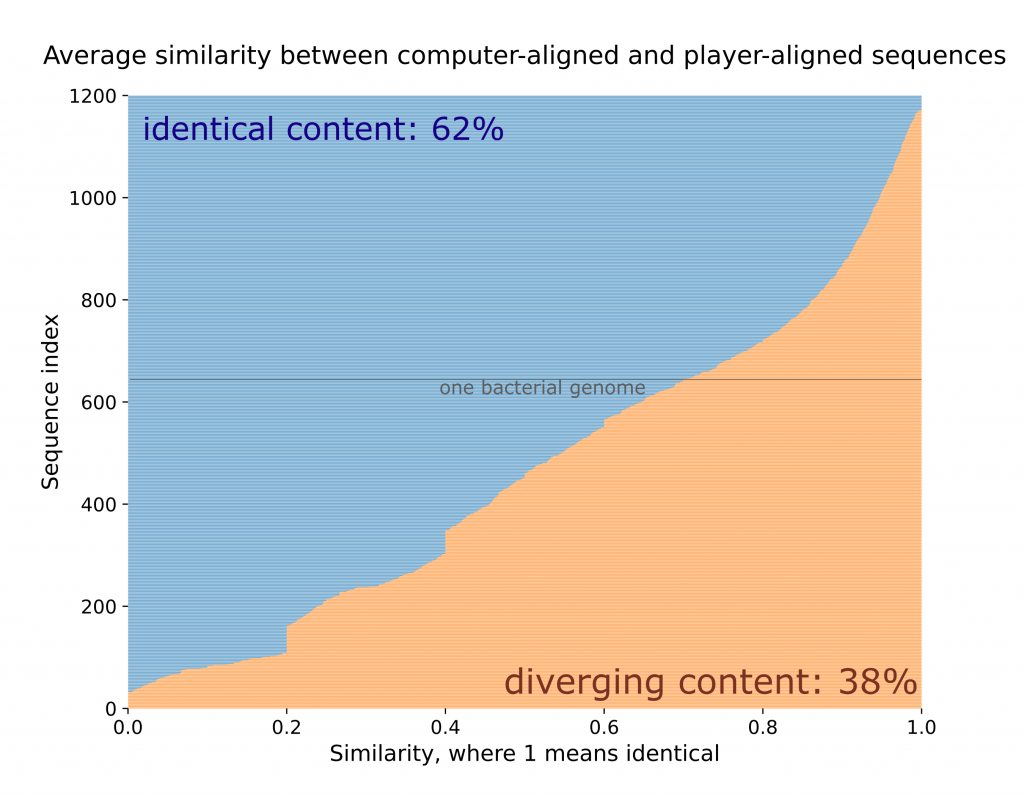
HOW MUCH DO PLAYERS’ SOLUTIONS DIFFER FROM EACH OTHER?
That is the next logical question. It is good that the average solution is different from the computer’s, but we will be able to learn a lot more if those solutions show a healthy dose of divergence. In short, if all player solutions are identical, there is probably an issue in the design of the puzzle because that does not take advantage of the large scale aspect of this community-oriented project; there is no upside to getting one hundred thousand identical solutions vs getting one hundred identical solutions. On the other hand, you wouldn’t want all the solutions to be distinct either, because then this would mean there is no signal, and that humans cannot arrive to a consensus in solving that problem. The hope is for solutions that are different but not too different. To give you a taste of what the results look like in that regard, we selected 100 random sequences of the same small region (5 nucleotides) of the genomes. For each sequence, we went through all the puzzles in which it was featured, and compared all the players’ solutions together, to assign average similarity scores. In this context, a similarity of 1 would mean the bricks were placed in the same positions through all puzzles featuring this sequence.
We show those results in the following heat map, where each little square is a nucleotide, and its color encodes the average similarity between all player-submitted sequences for this specific position. Each column shows 25 of the 100 sequences. You can see that some rows are fully dark blue (i.e. all occurrences in the puzzles are placed identically), but many have some light blue. Overall, 30% of solutions show a mean similarity under 80% which matches our objectives in terms of the trade-off between answer variety vs consistency.
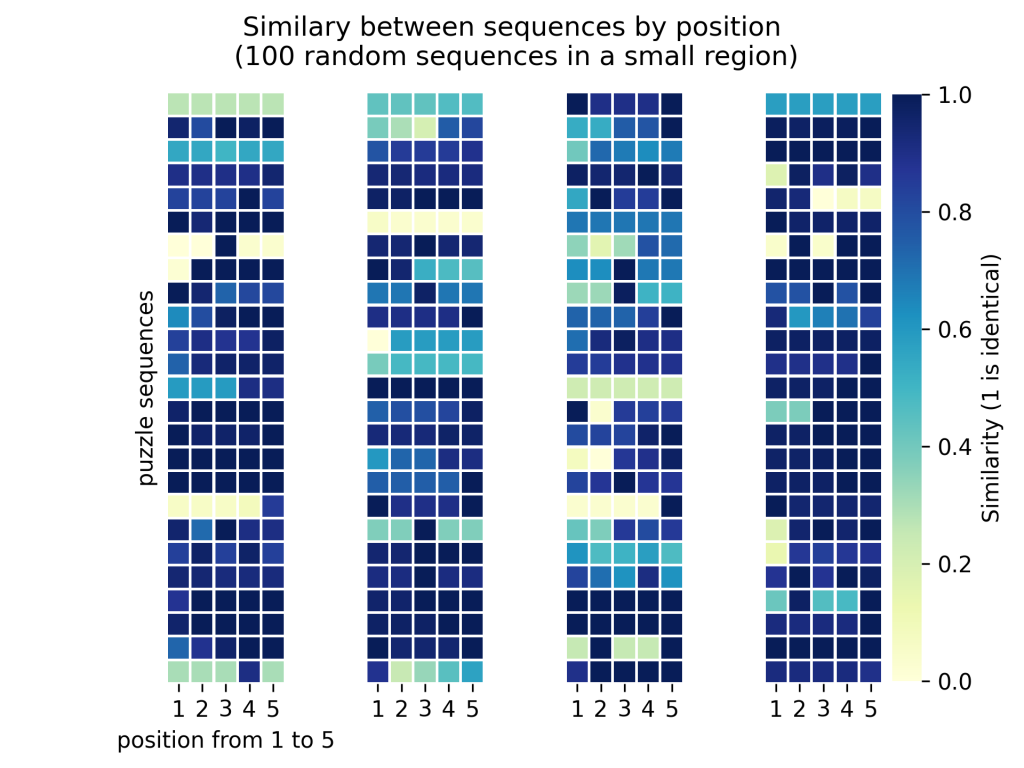
So how close are we to being done solving the problem?
That is a good question! First, we want to clarify that we will never run out of science to do; once we are done with the initial set of bacterial DNA sequences we were working on, we will generate puzzles with new sequences to solve other, related problems. In terms of the initial problem described in the presentation video, things are looking great! We have now completed roughly 80% of Phase 1. Phase 1 aimed at achieving a good coverage of the initial alignment, by pumping out many reasonably simple puzzles to a large number of players to gather a lot of simple data. We have generated a plot to show how much of the total alignment the players have analyzed so far. On this plot, like on the first one of this post, each horizontal line is a bacterial genome, and each vertical line is a position of the sequence (what you see as a row in the puzzle). Unlike in previous plots, all positions of the alignment are shown. Each little horizontal red line is a fragment of sequence that appeared as a column in at least one puzzle solved by 50 players. Here, keep in mind there is a lot of redundancy within regions, so the goal to obtain a good alignment is not to achieve a fully red square, but instead to have some red in every region of the square. Then, we can use the information displayed in red to re-align the adjacent nucleotides. As you see, we (ahem, you!) are doing pretty well for ourselves!
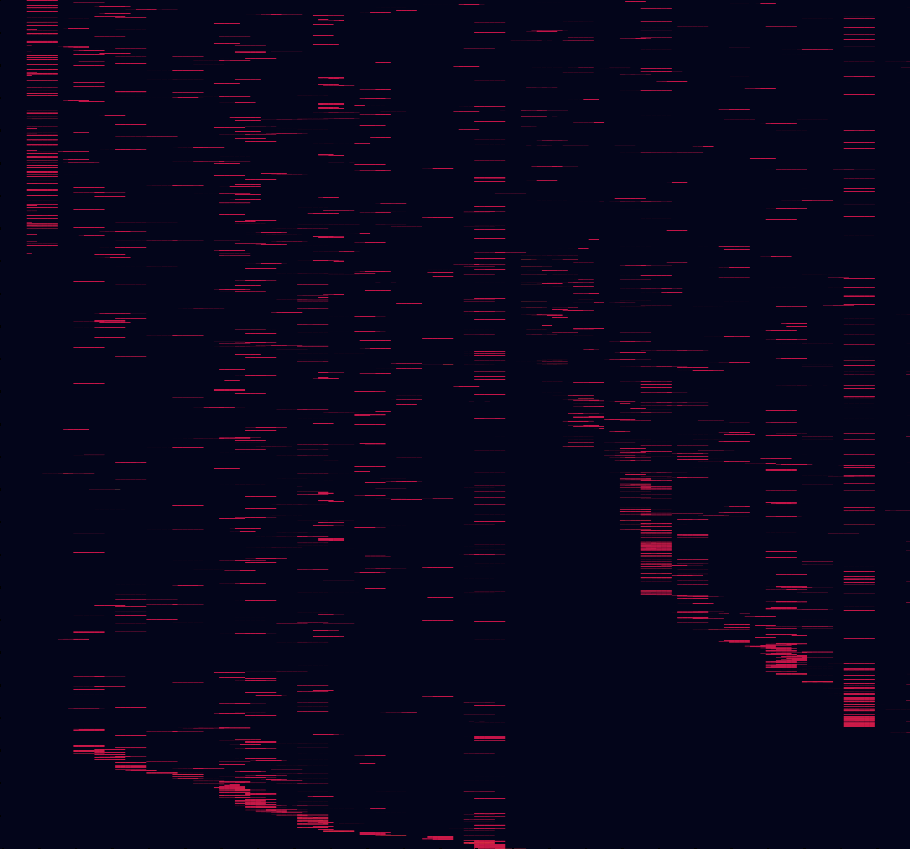
The analysis of this data will allow us to assess the performance of the players in the context of the alignment, identify regions for which this basic data is sufficient to achieve a good alignment (some regions are easier to align than others), and identify target regions for Phase 2. In Phase 2, we will be releasing more complex puzzles, with slightly more bricks and sometimes slightly more rows and columns. Moreover, we will be adding many puzzles with player-generated target scores, which will be significantly more challenging than computer-generated target scores at higher levels. This will allow us to build on the results of previously completed puzzles, by seeing if other players can do better, or how much improvement to a solution that looks good can be achieved by adding a couple yellow tokens (or gaps). And on your side, it will allow returning players to find enjoyment in more challenging puzzles now that they are acquainted with the game.
We estimate that to start Phase 2, we need to have ~40 million puzzles completed, and as soon as we reach that objective, you should stay tuned for some puzzle updates! We will keep you posted via this website, reddit and Twitter.